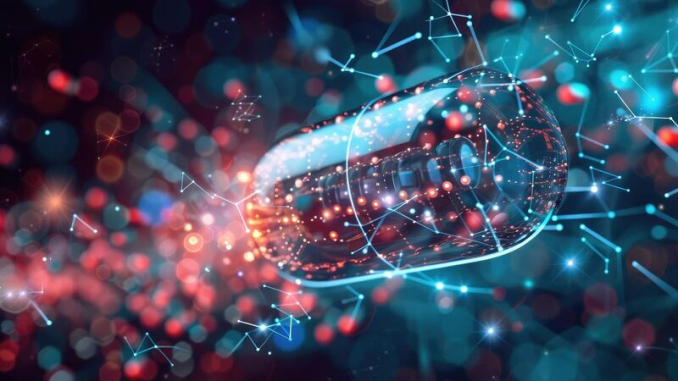
Because of the appearance of artificial intelligence (AI) in recent years, some of the methods by which drug discovery is approached have already changed fundamentally.The lengthy and costly process of drug development has over the years resulted in steep prices for the most popular medications. Within OTC whole business model, it costs billions of dollars a decade before a new can that has passed every safety and quality check is ready to be sold anywhere on earth. In other words, its high prices leave people little choice but to turn themselves into refugees from the impoverished Western world.But AI promises to speed up studies, reduce costs — and maybe even get life-saving medicine on the market sooner. This feature examines in many places how AI is changing us in depth on page thirty led?”The Rose. ” (The future is when drug discovery done by AI will be like a shooting star in dye, the heavier it is tinted before being sent over to computer interface hands and watched until it fades into nothingness on your screen.)
Traditional Drug Discovery: Hurdles and Needs a Change in Approach
This process is enough trouble. Until a drug is finally put on the market, the traditional steps are: Target identification: In this stage, scientists must find out the biological targets (such as proteins or gene) responsible for various diseases. Lead discovery: After a target is identified, researchers in laboratories both industrial and university set up thousands or even millions of screens with compounds, in the hope that they will find one able to interact with their discovered target.
Preclinical and clinical testing: When drug candidates with potential “candidates” are discovered, R&D labs and clinical trials begin a whole programme of rigorous testing into their safety effectiveness. At every stage, failure reigns supreme. Preclinical screening My tests just take too long and use much human labor, CTMS told me that. As a result, they rapidly filter out for further research or testing these very promising drug candidates–with high rates of attrition, long periods between failures and heavy financial losses to the pharmaceutical companies concerned.
AI and Drug Discovery: Revolutionizing Our Approach
That’s what AI is all about. Using machine-learning (ML) algorithms coupled with advanced computational models, AI can greatly speed up every stage of drug discovery. This is how AI is changing the game:
My schedule is too cramped to turn this into an immutable daily action, but as an example just after the finish of shots emptied source files A.
More Rapid Target Finding
AI can run through large data sets at speed and uncover what drugs might serve as targets — a task that combines gene analytics and proteomics with clinical data. Complex biological networks can be analyzed through this technique and connections identified. It is possible to determine at a much earlier stage than human beings can manage which genes are involved with specific diseases using this network based systemic analysis of all things at once: For example, with AI algorithms based on patient data predicting how disease processes, it is possible to home in on related targets for treatment in a more timely way.
This increases the chances of getting drugs that will be effective on the specific patient groups involved.
Speeding Up Lead Discovery and Compound Screening
In traditional drug development, chemical libraries of thousands upon thousands molecules are screened by laboratory analysis for new leads. Slowly and expensively. AI can shorten this work to virtual screening: through computer simulations that process known biological activity information, it helps determine any drug-like compounds which appear promising. Machine learning models use objective methods to predict the behavior of different molecules at specific drug targets.
Furthermore, AI enables today’s researchers to model and simulate chemical reactions. That means predicting how molecules actually behave without having to test each compound individually. AI-driven platforms such as DeepMind’s AlphaFold are already making great progress in this area. They are able to accurately forecast the shape of proteins — a key requisite for understanding how drugs interact with their targets.To add insult to injury, AI models that generate data rather than take in data have also come into being. These deep learning based systems dish up hundreds or thousands of potential drug molecules which, unlike the libraries today, do not yet exist, thus broadening the scope of candidates.
Preclinical Tests and the Design of Clinical Trials: A Support to Improve One of the major tasks of drug development is to show that a prospective medicine is both safe and effective for animals before it enters into human beings. Frankly, many drug candidates which look promising fail in clinical trials because they have unexpected side effects or simply do not work. This is where AI can help out by bringing greater accuracy into preclinical tests and d. changing widespread clinical practice as well.
Preclinical Tests and the Design of Clinical Trials: A Support to Improve One of the major tasks of drug development is to show that a prospective medicine is both safe and effective for animals before it enters into human beings. Frankly, many drug candidates which look promising fail in clinical trials because they have unexpected side effects or simply do not work. This is where AI can help out by bringing greater accuracy into preclinical tests and d. changing widespread clinical practice as well.
Preclinical Tests and the Design of Clinical Trials: A Support to Improve One of the main challenges in drug development is for a candidate medicine to be both safe and effective in human beings. Several drug candidates which appear promising never make it through clinical trials because they are either not effective or have unforeseen side effects. AI can help solve this problem by raising the accuracy of preclinical tests and one of its most common clinical d. rubus canescens eames—the design of trials themselves.
By AI guidance, predictive models were built upon historical clinical patient medical records and the genomic data of patients. For instance, it can predict how different populations at much level and moving into New England from there will react to new drugs, saving China up till then the future trial expenses plus any number of failure cases that might arise. This means, for pharmaceutical companies, far stronger confidence in proof–and faster regulatory reviews too.
Real World Outcomes of AI Driven Projects: Stories of Success in Drug Discovery and As AI was not seen independently in its latest phase of development. The dogmatic cheerleaders for drug discovery and life sciences have had many a success story which stand out. AI does have its limitations, however. But Exscientia is a UK-based company that has developed a new compound for Obsessive Compulsive Disorder (OCD) sufferers. It went from design stage through human trials in record time–and did so a final order of magnitude more rapidly than either traditional drug discovery or even Japan’s high – speed approach to discovery would allow! Talking about how the company was applying new principles in drug discovery, he explained that Exscientia–using AI–turned an initial design into actual clinical trials for patients within 12 months, whereas the normal amount of time it would take is five years or more.
In biotech, Insilico Medicine is a company that used AI to find a new drug candidate for fibrosis in just 18 months (that otherwise takes four to six years). Atomwise is using its platform technology, based on structure-based drug design guided by AI, with various research institutions to speed up drug discoveries against diseases such as Ebola, multiple sclerosis and leukemia. All three examples demonstrate that AI can significantly shorten the timetable of drug development even if its specificity makes it more efficient.
Challenges and Ethical Questions
While AI is transforming drug discovery, it will bring special challenges and ethical questions too. Data Privacy and Bias: AI models need huge bodies of input data. Its release for medical purposes inevitably stirs up major security concerns about how to handle such information. The bias in training data sets may produce unfair predictions. As a result, a drug may turn out to have little effect for certain populations.
Drawing robots are the best choice for those companies operating under those circumstances in need of such drugs. At the same time though, the advantages of making use this form of Manufacturers supplied medicines for when laboratory lies not in dependence on huge initial investment and ready resources but rather its convenience and flexibility to allow a variety medical treatments available just around corner.
Robots have remove triazine from world of formaline. Drugs general in modern usage: Nerve gas-KS 6 and crack-cocaine There are many kinds with corresponding different mechanisms or targets… One compound is useless for all purposes The changed form can be given different chemical names depending upon its properties. Therefore it certainly looks well worth develop-Ing drugs kindly for human consumption.
Transparency and Interpretability: Many AI algorithms, especially those using deep learning techniques, are often called “black boxes” because of the lack of transparency in how they make decisions. For AI to be widely accepted in drug discovery, besides making recommendations from an administrative body regulate such research activities, the scientific community must also understand how these systems reach conclusions.
The Future of AI in Drug Discovery
AI in drug discovery looks promising—Conceived in a moment that gave birth to such techniques as quantum computing and multi-omics (integration of genomics, proteomics and other biological data). Also, through collaborations between pharmaceutical companies, start-up AIs and academic institutions will push AI in directions as yet unforeseen in this area.
AI can create drugs according to the unique genetic and molecular makeup of each individual. Along these lines, thanks to its unusual ability to handle huge data sets and make accurate predictions of future developments, AI has the potential not only to greatly shorten the period required for developing newly discovered drugs but also revolutionize everything about pharmaceutical industry.
Conclusion
AI is making increasingly great contributions to the discovery of new drugs, speeding up development and reducing costs. It is all part of the drug development structure. There remains obstacles to overcome but huge potential rewards are waiting—profound changes that could reform most pharmaceutical landscapes and help patients receive long-needed therapies. And as AI continues to evolve, its role in the pharmaceutical R & D process can only become more deeply entrenched—pushing the boundaries of what we now call “modern medicine” farther back yet again.
Leave a Reply