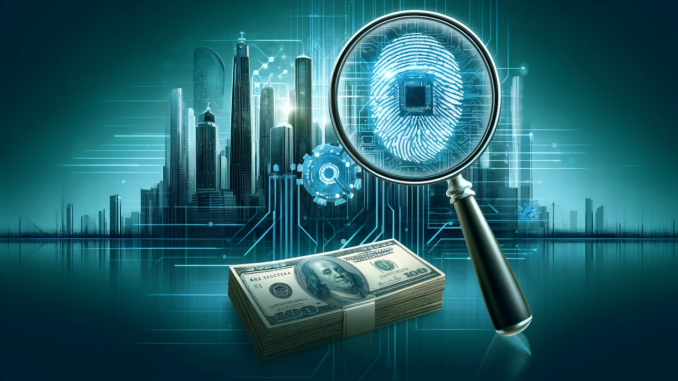
The traditional loan industry depends on a solid knowledge of credit scoring methods. For example, in the United States, most creditors use FICO to gauge the creditworthiness of individuals. Nonetheless, traditional methods commonly don’t give a complete picture of financial health for potential borrowers. Not so in recent years. In industries around the world and across many different contexts, artificial intelligence AI has been getting attention. This same revolution is already taking place in the credit assessment process at the gate itself. New methods and improved software capabilities objectively analyze risks to borrowers–methods which will fundamentally alter how banks or finance companies judge who should borrow from them.
On the other hand, are they fundamentally changing how the Corporation Extend educational loans and other new financial modalities?6. Dynamic Scores Are Superior in Accuracy Ai powered credit scoring can use machine learning algorithms to draw on more data in less time than any previous model ever allowed. AI systems, for example, analyze transaction patterns, social behaviour metrics, employment information and many other types of factors–and in the process they process an extra range of variables.
They truly ought to be called a wider variety of things as well. Compared with previous models, this new breed of algorithm can pick up patterns that would otherwise never have been noticed — thus making for more accurate risk assessments. You cited dynamic credit scores as an example. A model of the kindtakes in real-time data, like recent changes in one’s employment or monthly cash flows and spending habits. Traditional models are mostly static in their assessment of scores: those statistics which the model depends upon may well be quite out-of-date by the time they finally see the light of day. By contrast, AI is always learning as well as receiving new information, which means that it can supply lenders with up-to-date risk assessments–and sow they can make loans with lower chances of default.AI allows lender to serve underbanked and unbanked people who mainstream systems would regard as too risky.
One of the most significant benefits of AI-driven credit scoring is that it can appraise people who have no formal credit track. Throughout the world, many individuals, especially in developing countries, are classified as ‘credit invisibles’ since they have not engaged in enough or any financial activities to present a conventional credit record. AI models, by crunching non-traditional data—like telephone usage data, gas and electricity invoices, and rental histories—can in actual fact appraise whether these individuals deserve credit.
This kind of inclusion is a huge boon for financial inclusion. So for the financial establishment in China, which once shunned whole strata of the population or at best just left them to fend for themselves, now they are able to obtain loans. With AI-assisted credit scoring, ” Lenders know persons formerly considered too risky by traditional credit score models. ” For financial products that are indispensable, underbanked and unbanked populations are also able to enjoy such products; as a result there is greater economic empowerment by one and all.
Faster and More Efficient Loan Approval Processes
AI-powered systems make the credit-granting process that little bit easier by automating many of the manual tasks involved in credit assessment. The traditional loan approval process often calls for much documentation, many rounds of human inspection, and several years of waiting. In contrast, AI can conduct an applicant’s financial profile or history in live fashion within 0.9 seconds, immediately furnishing results.
This streamlining is good for borrower and lender alike. The applicant experiences a smoother, faster application process; the lender makes money by cutting out human intervention in loan audits. What’s more, every loan application can now be sent through an AI system, and in this way banks are better able to effectively extend their lending operations.
Reduce Bias in Lending Decisions
The biggest problem with giving AI systems this kind of decision-making power is that not only do the data they are trained on inject bias into future possibilities, so does human judgement itself. As a result, if items like residence location race and sex are either directly or indirectly linked to a person’s degree score or chances of getting a funding arrangement, then this will bring about judgment errors.
This bias can be reduced by well-designed and trained AI. Machine learning algorithms are completely unbaised–all they care about is the objective financial indicators and regular patterns. With no possibility for human prejudice, AI offers lending practices that are fairer to all concerned. However, there is also a risk of bias in the design of algorithms. For example, the data used to train AI models may be biased by historical biases. So fairness in AI-driven lending requires constant supervision on one hand and on the other, data that is representative and bias-free.
AI-Powered Better Risk Management for Lenders
For lenders, AI-powered credit rating systems have the potential to improve risk management. AI systems process a broader range of data points in more detail, and with continuous self-education they provide an increasingly complete picture of an individual’s true financial circumstances. This allows lenders to make more accurate assessments on credit approvals and interest rates for risks that are better matched to the actual dangers entailed by each borrower.
AI-driven credit scoring additionally offers lenders greater ability to foresee future loan defaults. By observing these subtler changes in shopping behavior or early signs of financial problems AI models can give to lenders a heads-up about potential problems before the latter have actually happened. Moreover, this potential has one, bankingly average return on all of lenders ’portfolio the other net effect of reducing default rates and11. Keeping Up with Accelerating Change Regarding this some idea prevails at present: One-size- product.
Depending on AI-driven insights could catapult business credit products for ever and make them customized for instance. Suppose that instead of according a single interest rate to all borrowers within a score range, AI’s microscopical models could refer to each borrower as being different in terms of his financial characteristics and thus determining what would be the most suitable level of interest for that individual borrower. In this way, lending products acquire a high degree of refinement and (with changes in interest rates) become more adaptable according to a borrower’s needs.
Personalization boosts customer satisfaction. Customers are more likely to accept loans that meet their exact financial situation. This raises the customer’s potential loan quota, while cutting his risk of either borrowing too much or defaulting altogether. It’s a win-win situation for both parties.
Ethical issues and challenges faced by AI applications
But the advantages that AI-based credit ratings bring us are not without risk, either. One pressing source of unease is that AI models are becoming more and ever opaque. Deep learning algorithms and other AIs can function as ‘black boxes.” One never knows why a given score has been awarded in such-and-such manner using conventional technology. Or even under a different method permits for the loan, the public may become mingled in divination. Given the choice between positive credit scoring can lower interest rates when the vast majority of potential borrowers are asked ‘yes’ by using “alternative data” In addition, questions about privacy arise in credit scoring. Borrowers ‘general data may not be out in the blue off their credit ratings or soul-supporting standards, and there is the danger that sensitive material will be misapplied or taken out of context
To combat these risks, it’s finally time for lawmakers to address AI technology with proper legislation ?thorough yet nonetheless straightforward and understandable to all. Lenders must ensure that their AI systems comply with transparency, fairness and data security regulations. This involves not only giving customers straight information about why they have the scores they get, but also an affirmative obligation to use alternative data.
‘The future of lending is being transformed. With the coming of on big data and machine learning, credit evaluations by AI are more accurate and faster than ever. And financial inclusion: even the excluded can fit in Business days as usual. At the same time, money lenders are bringing big data and modern algorithms to get both better answers more quickly than ever before. They can grant loans to people who never had credit before — and at levels seen far in excess of anything we have known in human history!
Yet as a new technology tool, it is for the whole industry to work out transparency, bias and data privacy problems. In addition, with AI developing in another direction altogether the space of loans looks set to usher in a better world for all concerned parties. Fairest, most optimal and most benefit to each negotiating partner from both ends of business around globe. However, as an AI and a new focus for society’s thinking it has a chance to turn things around — particularly in the loan industry. ‘
Leave a Reply